For small data machine learning in materials science, tools and software like Python, Jupyter Notebook, scikit-learn, and TensorFlow are commonly used. These tools provide efficient and user-friendly platforms for data analysis and model development, allowing for valuable insights to be gained from limited datasets.
In the rapidly evolving field of materials science, the utilization of machine learning tools and software has become increasingly crucial for making sense of small datasets. Python, with its extensive libraries for data analysis and visualization, is a popular choice for researchers in this field.
Jupyter Notebook provides an interactive platform for code execution, further facilitating the exploration of small datasets. Additionally, scikit-learn offers a range of machine learning algorithms and tools, while TensorFlow enables the development of neural network models, making it easier to extract meaningful insights and patterns from limited data. These tools and software collectively provide a robust foundation for unlocking the potential of small data machine learning in materials science.
Data Collection And Preprocessing
Small data machine learning in materials science requires the use of specific tools and software to effectively collect and preprocess data. The process of data collection and preprocessing is crucial for obtaining reliable and accurate results in materials science research. In this section, we will explore the key aspects of data acquisition and preprocessing techniques for small data in machine learning for materials science applications.
Data Acquisition
When conducting small data machine learning projects in materials science, it is essential to carefully consider the methods of data acquisition. Datasets in materials science are often limited in size, making it crucial to capture all relevant information. The following strategies can be utilized for effective data acquisition:
- Collaboration with research institutions and industry partners for data sharing.
- Sensor technology for real-time data collection in material testing and analysis.
- Manual data collection from experiments and trials to ensure comprehensive dataset inclusion.
Preprocessing Techniques For Small Data
Preprocessing techniques play a vital role in preparing small data for machine learning analysis. In materials science, the following methods can be employed to preprocess small datasets effectively:
- Data Cleaning: Removal of outliers and irrelevant data points to enhance the quality of the dataset.
- Feature Scaling: Normalizing or standardizing features to ensure consistency in data representation.
- Dimensionality Reduction: Utilizing techniques such as principal component analysis (PCA) to reduce the number of features while retaining essential information.
- Imputation: Handling missing data through methods like mean or median imputation to maintain dataset completeness.
Feature Selection And Engineering
Feature selection and engineering play a crucial role in the field of small data machine learning in materials science. These activities are essential for identifying and creating meaningful, relevant, and informative input features that can significantly impact the predictive performance of machine learning models.
Importance Of Feature Selection
Effective feature selection is vital as it allows for the identification of the most influential attributes or variables that contribute to the predictive power of a model. By removing irrelevant or redundant features, the model’s complexity is reduced, leading to improved generalization and interpretability.
Techniques For Feature Engineering
Feature engineering involves creating new features from existing ones or transforming the existing features to better represent the underlying problem to the predictive models. Some common techniques include:
- Polynomial features
- Logarithmic transformation
- Bin counting
- Interaction features
Machine Learning Models
Machine Learning Models play a crucial role in the field of materials science. Leveraging these models, researchers can analyze small data sets more effectively, leading to valuable insights and developments in the industry. Let’s delve into the types of machine learning models commonly used in materials science and their significance.
Traditional Models For Small Data
In materials science, traditional machine learning models such as linear regression, decision trees, and support vector machines are frequently utilized to handle small data sets. These models allow researchers to extract patterns and relationships from limited data, enabling them to make informed decisions and predictions.
Advanced Models For Materials Science
Advanced machine learning models tailored for materials science, including random forests, neural networks, and deep learning, are becoming increasingly prevalent. These models offer superior accuracy and performance when working with small-scale data, empowering scientists to uncover intricate material properties and behaviors.
Evaluation Metrics
Evaluation metrics are crucial for assessing the performance of machine learning models in materials science. These metrics provide valuable insights into the accuracy, precision, and efficiency of the models, helping to determine their effectiveness in solving specific material-related challenges.
Key Metrics For Model Evaluation
When evaluating machine learning models for materials science, it’s essential to consider a range of key metrics to gauge their performance. These metrics include:
- Accuracy: Measures the overall correctness of the model’s predictions.
- Precision: Indicates the proportion of true positive predictions out of all positive predictions made by the model.
- Recall: Reflects the ability of the model to identify all relevant instances in the dataset.
- F1 Score: Harmonic mean of precision and recall, offering a balanced assessment of the model’s performance.
- Mean Absolute Error (MAE): Measures the average absolute differences between predicted and actual values.
- Mean Squared Error (MSE): Calculates the average of the squares of the errors between predicted and actual values.
- R-squared: Indicates the proportion of the variance in the dependent variable that is predictable from the independent variables.
Choosing The Right Metrics For Materials Science
For materials science applications, it’s crucial to choose the most appropriate evaluation metrics based on the specific goals and characteristics of the ML model. For instance, when dealing with material property prediction, metrics such as MAE and MSE are particularly important for assessing the accuracy of predictions. On the other hand, in classification tasks related to material recognition or categorization, precision, recall, and F1 score play a significant role in evaluating the model’s effectiveness.
Tools For Small Data Machine Learning
When it comes to small data machine learning in materials science, having the right tools and software is crucial. These resources enable researchers and scientists to extract meaningful insights from limited datasets. In this blog post, we’ll explore the essential tools and software for small data machine learning in materials science, focusing on Python libraries and commercial software tailored for this specific field.
Python Libraries For Small Data
Python libraries are indispensable resources for conducting machine learning tasks, especially when dealing with limited datasets in materials science. These libraries offer a wide range of functions and algorithms designed to effectively handle small data and extract valuable information from it. Some key Python libraries for small data machine learning in materials science include:
- scikit-learn: A versatile library that provides various algorithms for classification, regression, clustering, and dimensionality reduction, making it suitable for small data analysis.
- TensorFlow: Known for its flexibility and scalability, TensorFlow offers tools for building and training machine learning models with small datasets, particularly beneficial for materials science applications.
- PyTorch: This library is widely used for its dynamic computational graph and efficient handling of small dataset training, delivering high-performance results for materials science machine learning tasks.
Commercial Software For Materials Science
Apart from open-source Python libraries, commercial software tailored for materials science plays a crucial role in small data machine learning. These software solutions offer advanced functionalities, dedicated support, and user-friendly interfaces, catering specifically to the unique challenges within this domain. Some notable commercial software for small data machine learning in materials science are:
- Materials Studio: A comprehensive software package that provides an extensive range of tools for modeling and simulation, including machine learning capabilities for small datasets in materials science.
- CrystalMaker: Recognized for its powerful visualization and analysis features, CrystalMaker offers machine learning functionalities tailored to handle small data in materials science research.
- ASE: Atomic Simulation Environment: While primarily a Python library, ASE also offers commercial support, delivering specialized machine learning modules designed for small dataset challenges in materials science.
By leveraging these Python libraries and commercial software, researchers and scientists can effectively harness the power of small data machine learning, unlocking valuable insights and advancements in the field of materials science.
Software For Materials Science
In the field of Materials Science, software plays a crucial role in enabling scientists and researchers to analyze, simulate, and model various properties and behaviors of materials. This software enhances the capabilities of small data machine learning, allowing for more efficient and accurate predictions and analyses. Let’s explore some of the key software tools utilized in Materials Science for small data machine learning applications.
Instrument Simulation
Instrument simulation software in Materials Science enables users to replicate and simulate the behavior of various instrumental techniques used for material characterization. These tools allow scientists to understand the potential outcomes of specific experiments, aiding in the design of experiments for collecting the necessary small datasets. By simulating the performance of analytical instruments, researchers can optimize their experimental setups and data collection methods, ultimately leading to improved machine learning models. Popular examples include software for simulating X-ray diffraction, scanning electron microscopy, and atomic force microscopy.
Molecular Dynamics Software
Molecular dynamics software is essential for studying the dynamic behavior of atoms and molecules within materials. This type of software leverages algorithms and computational methods to simulate the movement and interactions of atoms over time, providing valuable insights into the properties and performance of materials at the atomic level. By utilizing molecular dynamics software, researchers can generate small datasets related to material structure, behavior, and properties, which can then be used for machine learning applications to make predictions and analyze material characteristics. Examples of molecular dynamics software include LAMMPS, GROMACS, and NAMD.
Case Studies
Case studies are an excellent way to gain insights into the practical applications and successful implementations of Small Data Machine Learning (ML) in the field of Materials Science. By examining real-world scenarios, we can better understand the impact of ML tools and software on enhancing the development and analysis of materials. In this section, we will explore the application of Small Data ML in Materials Science and highlight successful case studies where ML tools have made a tangible difference.
Application Of Small Data Ml In Materials Science
- Efficient prediction of material properties with limited datasets
- Identification of relationships between material structure and performance
- Accelerating materials discovery through targeted data analysis
Successful Implementations Of Machine Learning Tools
- Improved prediction accuracy of mechanical properties using ML algorithms
- Optimization of material synthesis processes based on ML-driven models
- Enhanced material classification and identification through machine learning techniques
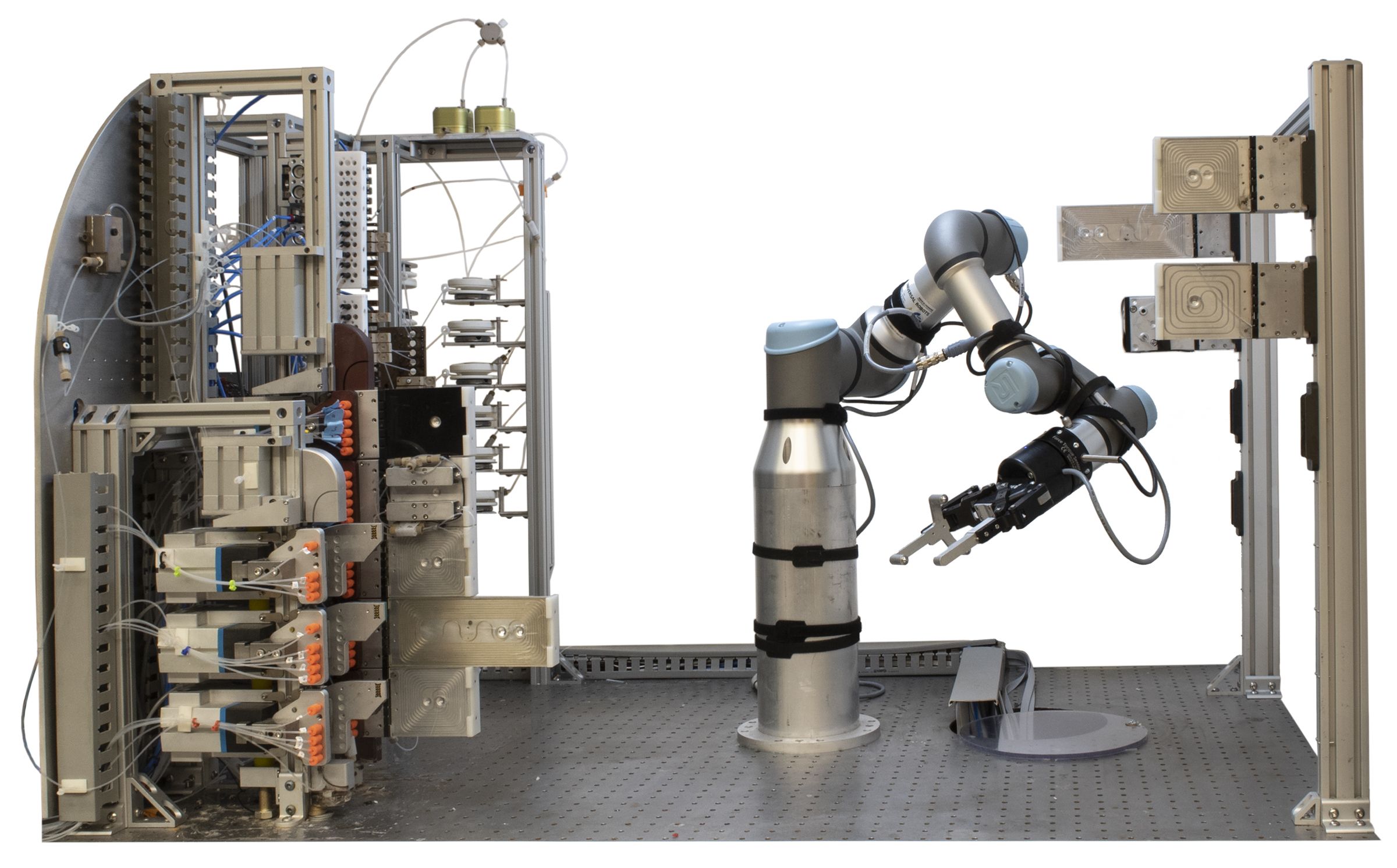
Credit: news.mit.edu
Challenges And Future Prospects
Small data machine learning in materials science presents several challenges, but its future prospects are promising. Overcoming the limitations and obstacles faced by researchers and developers is crucial for advancing this field. The future developments and opportunities in small data machine learning have the potential to revolutionize materials science research, leading to significant breakthroughs and innovations.
In small data machine learning for materials science, researchers encounter various limitations and obstacles that hinder the process of generating accurate and reliable models. Some of the primary challenges include:
- Limited dataset sizes
- Noisy and heterogeneous data
- Complex feature selection
- Overfitting due to small sample sizes
- High-dimensional data
Addressing these limitations and obstacles is essential to improve the efficacy of small data machine learning techniques in materials science.
In the future, advancements in small data machine learning in materials science are poised to bring about significant opportunities. Some potential developments and opportunities include:
- Integration of domain knowledge to supplement small datasets
- Refinement of algorithms for small data scenarios
- Application of transfer learning techniques
- Utilization of Bayesian methods for uncertainty quantification
- Exploration of active learning approaches for efficient data collection
These future developments and opportunities hold the promise of enhancing the capabilities of small data machine learning in materials science, opening doors to new discoveries and advancements in the field.
Conclusion
Incorporating the right tools and software is crucial for small data machine learning in materials science. By adopting these technologies, researchers and scientists can enhance their data analysis and modeling capabilities. This enables them to make more accurate predictions and drive innovation in the field of materials science.
Embracing these resources is key to staying ahead in this dynamic and competitive industry.