Using machine learning techniques for small data in materials science requires a tailored approach to compensate for the limited data volume. With small datasets, techniques like transfer learning, semi-supervised learning, or Bayesian methods can yield accurate results in materials science research.
Machine learning models designed for small data can effectively uncover valuable insights, aiding in the development of new materials and understanding material properties. Small data presents a unique challenge in materials science research, where traditional machine learning models may struggle to provide accurate predictions.
However, implementing specialized techniques tailored for small datasets can unlock the potential for groundbreaking discoveries in material properties and structure-function relationships. By harnessing the power of transfer learning, semi-supervised learning, or Bayesian methods, researchers can efficiently leverage small data to drive advancements in materials science. This not only accelerates the development of new materials but also enhances the understanding of existing materials, propelling scientific innovation in the field.
Understanding Small Data In Materials Science
Materials science involves studying the properties and behaviors of various substances to understand their potential applications. In the context of machine learning, the availability of small data sets in materials science presents unique challenges and opportunities. Understanding the specific considerations related to small data sets in this field is crucial for the development of effective machine learning techniques.
Definition And Significance
Small data sets in materials science refer to relatively limited amounts of experimental or observational data available for analysis. Due to the inherent complexities and costs associated with data collection in materials science, it is common for researchers to work with small data sets. The significance of understanding small data lies in the need to develop machine learning techniques that can extract meaningful insights from such limited sources of information, ultimately contributing to advancements in material design and development.
Challenges And Limitations
The challenges and limitations of working with small data in materials science are multifaceted. These include issues such as limited sample sizes, high-dimensional feature spaces, and the need for specialized feature extraction methods to capture the nuances of material properties. Furthermore, the inherent variability and noise in small data sets can pose significant hurdles for traditional machine learning algorithms, requiring the development of tailored approaches to address these challenges.
Importance Of Machine Learning In Materials Science
Machine learning has proven to be a pivotal technology in the field of materials science, revolutionizing the way small data sets are analyzed and the insights they yield. The ability of machine learning techniques to process, interpret, and derive patterns from data has opened new frontiers in materials science research, propelling the field towards greater efficiency, accuracy, and innovation.
Enhancing Data Analysis
Machine learning techniques offer a powerful means of enhancing data analysis in materials science. By employing sophisticated algorithms and models, researchers can gain deeper insights into the behavior, properties, and performance of materials, even with limited data. The use of machine learning tools enables the extraction of complex patterns and correlations, leading to a more comprehensive understanding of the underlying structures within the data.
Leveraging Small Data For Insights
Machine learning in materials science allows for the effective leverage of small data to derive valuable insights. Through advanced predictive modeling and feature engineering, machine learning algorithms can uncover significant trends and relationships within small data sets, yielding crucial information for material design, optimization, and discovery processes. This capability to extract meaningful insights from limited data has revolutionized the way researchers approach challenges in materials science, paving the way for breakthrough innovations.
Data Preprocessing In Materials Science
Data preprocessing in materials science is a crucial step in leveraging machine learning techniques for small data. It involves the manipulation and preparation of data to ensure its suitability for analysis and modeling. By focusing on data cleaning and formatting, as well as feature selection and engineering, researchers can optimize the potential of their small datasets for meaningful analysis.
Data Cleaning And Formatting
Before delving into the analysis, it’s essential to clean and format the data for accuracy and consistency. This stage entails identifying and rectifying any errors, outliers, or missing values that could affect the integrity of the dataset. Data cleaning involves techniques such as imputation for missing values and outlier detection, ensuring that the dataset is robust and high-quality.
Feature Selection And Engineering
Feature selection and engineering focus on identifying the most relevant variables and creating new features to enhance the predictive power of the model. This involves selecting the most informative features to maximize the model’s performance while reducing the dimensionality of the dataset. Additionally, feature engineering entails creating new features or transforming existing ones to improve the model’s ability to capture underlying patterns in the data.
Machine Learning Models For Small Data
When it comes to materials science, the amount of available data can often be limited, posing a significant challenge for traditional machine learning models. Fortunately, there are specific machine learning techniques tailored to effectively handle small data sets in materials science. These techniques, when harnessed the right way, can unlock valuable insights and patterns that may have otherwise been obscured by the limitations of small data. In this blog post, we will delve into the machine learning models designed to address the unique demands of small data in the context of materials science.
Supervised Learning Techniques
Supervised learning techniques entail training a model using labeled data. In the context of materials science, supervised learning can be utilized to predict material properties, classify various materials, and optimize material synthesis processes. Some popular supervised learning algorithms for small data include:
- Decision Trees
- Random Forest
- Support Vector Machines
- Neural Networks
Unsupervised Learning Techniques
Unsupervised learning techniques are invaluable for small data in materials science, as they can reveal hidden patterns and structures within the data. These techniques are often utilized for clustering similar materials, dimensionality reduction, and identifying outliers. Common unsupervised learning algorithms suitable for small data include:
- k-Means Clustering
- Principal Component Analysis (PCA)
- t-Distributed Stochastic Neighbor Embedding (t-SNE)
- Self-Organizing Maps (SOM)
Semi-supervised Learning Techniques
Semi-supervised learning techniques offer a middle ground between supervised and unsupervised learning, leveraging a small amount of labeled data and a larger pool of unlabeled data. In materials science, this approach can be particularly beneficial when labeled data is scarce. Some effective semi-supervised learning techniques for small data include:
- Label Propagation
- Self-Training
- Co-Training
- Generative Adversarial Networks (GANs)
Overcoming Challenges In Small Data Analysis
Small data analysis in materials science poses unique challenges due to the limited amount of data available for analysis. However, there are several machine learning techniques that can be leveraged to overcome these challenges and extract meaningful insights from small datasets.
Transfer Learning
Transfer learning, a powerful machine learning technique, can be particularly useful for small data analysis in materials science. This approach involves leveraging knowledge from a pre-trained model on a larger dataset and fine-tuning it for the specific small dataset at hand. By transferring knowledge from a related domain or larger dataset, transfer learning can help mitigate the limitations of small data and improve the performance of machine learning models.
Active Learning Approaches
Active learning approaches are another effective strategy for optimizing small data analysis in materials science. By selectively labeling the most informative instances in the dataset, active learning allows the model to iteratively acquire new data points, thereby improving its performance with minimal labeled data. This iterative process of selecting the most valuable data points for labeling can significantly enhance the efficiency and accuracy of machine learning models in the context of limited data.
Model Validation And Evaluation
Model validation and evaluation are crucial steps in the process of harnessing the power of machine learning techniques for small data in materials science. It is essential to ensure that the developed models are effective, accurate, and robust in making predictions and drawing insights from limited datasets. In this section, we will delve into the strategies and methodologies employed for validating and evaluating machine learning models tailored specifically for small data in materials science.
Cross-validation Techniques
When dealing with small datasets, cross-validation techniques play a pivotal role in ensuring the reliability and generalizability of machine learning models. K-Fold cross-validation is a widely used approach in which the dataset is partitioned into k subsets, and the model is trained and tested k times, with each subset serving as the testing set exactly once. This technique allows for robustly assessing the model’s performance and reducing the impact of data variability inherent in small datasets.
Evaluation Metrics
Evaluation metrics are instrumental in quantitatively measuring the performance of machine learning models for small data in materials science. Commonly used metrics such as mean squared error (MSE), mean absolute error (MAE), and R-squared (R2) provide insights into the accuracy and predictive capabilities of the models. Additionally, specific domain-centric metrics may be adopted to evaluate the model’s performance in addressing material science-related challenges, such as material classification accuracy, material property prediction accuracy, and feature importance scores. These metrics aid in identifying the strengths and limitations of the models, guiding further refinement and optimization.
Ethical Considerations In Small Data Analysis
When it comes to conducting small data analysis in the field of materials science, it’s crucial to consider the ethical implications that can arise. As the discipline increasingly relies on machine learning techniques to extract insights from limited datasets, ethical considerations play a pivotal role in guiding the handling and analysis of such data.
Bias And Fairness
One of the most critical ethical considerations in small data analysis is the potential for bias and fairness. Machine learning algorithms are susceptible to incorporating biases present in the data they are trained on, which can lead to skewed results. It’s imperative to address bias and ensure fairness to mitigate the impact of any prejudiced outcomes arising from the small dataset.
Privacy And Data Security
Considering privacy and data security when dealing with small data analysis is paramount. Ensuring that sensitive information is handled with utmost care and privacy regulations are adhered to is essential. Implementing robust security measures to safeguard the limited dataset from unauthorized access or breaches is a critical aspect of ethical small data analysis.
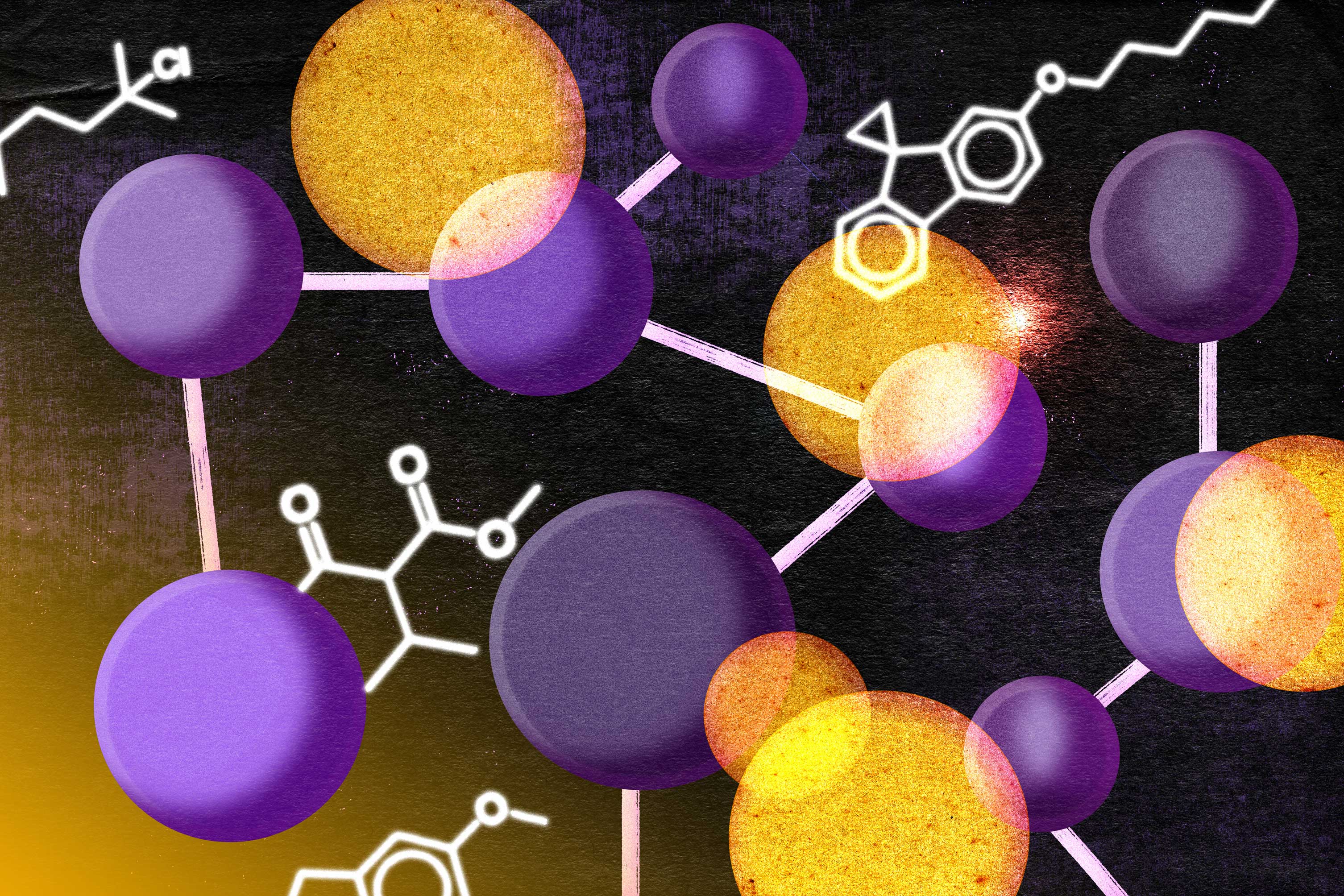
Credit: news.mit.edu
Future Prospects In Machine Learning For Small Data
In the field of materials science, as in many other scientific domains, collecting large datasets for machine learning applications is often impractical due to the high cost and time-consuming nature of data acquisition. However, the potential of machine learning techniques for small data in materials science holds a promising future.
Advancements In Data Augmentation
The use of data augmentation techniques has shown promising results in the field of materials science. By synthetically expanding the training dataset through techniques such as mirroring, rotating, and adding noise to existing data, machine learning algorithms can learn to generalize better and make accurate predictions even with limited data. Moreover, advancements in generating realistic synthetic data have opened new possibilities for training machine learning models on small datasets with improved performance, paving the way for greater applications in materials science.
Integration Of Domain Knowledge
Integrating domain knowledge into machine learning models has been a significant area of focus, especially in fields with limited data such as materials science. By incorporating expert knowledge about the physical and chemical properties of materials into the model architecture, the accuracy and interpretability of the models can be significantly enhanced. This approach not only helps in making better predictions with small datasets but also provides valuable insights to material scientists, thereby accelerating the discovery and development of new materials.
Conclusion
To sum up, when working with small data in materials science, machine learning techniques offer valuable insights and predictions. By leveraging the power of algorithms, researchers can derive meaningful conclusions and uncover patterns that may have been previously overlooked. As technology continues to advance, these methods will undoubtedly play a pivotal role in shaping the future of material science research.